Workflow Technologies Impact SC20 Gordon Bell COVID-19 Award Winner and Two of the Three Finalists
By Rob Farber, contributing writer
This year the winner and two of three finalists of the SC20 Gordon Bell Special Award for COVID-19 competition leveraged the scalable workflow technologies brought together by the ECP ExaWorks project. The ExaWorks team sees this as a demonstration of the effectiveness of custom high-performance workflows that use high-quality scalable workflow building blocks. At a high level, ExaWorks is building a Software Development Kit (SDK) for workflows and a community around common APIs and open-source functional components that can be leveraged individually, or in combination, to create sophisticated dynamic workflows that can leverage exascale-class supercomputers.
Shantenu Jha (Computation and Data-Driven Discovery Chair at Brookhaven National Laboratory) highlights the importance of the nascent ExaWorks SDK to the 2020 Gordon Bell COVID-19 award competition: “Long gone are the days when you can run a single application to get scientific insight. This is central as scientists now need to focus on workflows. The Gordon Bell awards are for insight. However, workflows are complex. All four COVID-19 award finalists involved workflows. Only one of the finalists used a pre-created workflow; the other three created workflows using components from the ExaWorks SDK.”
Long gone are the days when you can run a single application to get scientific insight. This is central as scientists now need to focus on workflows. The Gordon Bell awards are for insight. However, workflows are complex. All four COVID-19 award finalists involved workflows. Only one of the finalists used a pre-created workflow; the other three created workflows using components from the ExaWorks SDK — Shantenu Jha, Computation and Data-Driven Discovery Chair at Brookhaven National Laboratory
Referring to the ExaWorks SDK diagram in Figure 1, Jha continues, “Composability is a key aspect of the SDK. By composability, I mean selecting and bringing together these components to do science.” Jha notes that “components from the pink, blue, and purple parts of the ExaWorks toolbox were used in the Gordon Bell Finalist competition.” This article will discuss a number of the ExaWorks SDK components used in the SC20 competition.
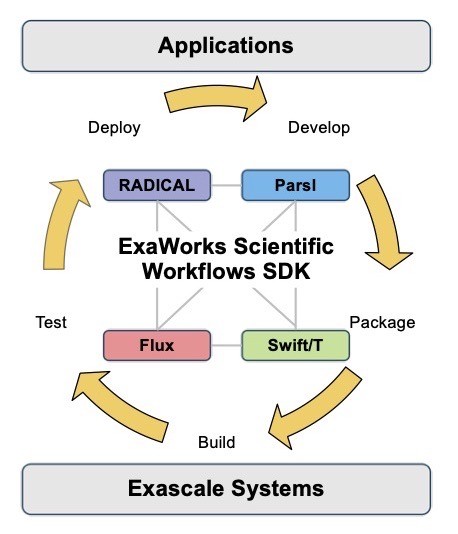
Figure 1: A schematic view of the ExaWorks SDK showing the initial seed technologies in pink, yellow, blue, and purple. (source: https://exaworks.org/)
The hidden work behind the amazing results
In discussing the incorporation of ExaWorks technologies into the workflows of the recent SC20 Gordon Bell Covid-19 submissions, Dan Laney, ExaWorks principal investigator (PI) and computer scientist at the Lawrence Livermore National Laboratory (LLNL) Center for Applied Scientific Computing, acknowledges the extraordinary science in the awards papers while pointing out that “Exaworks is focused on bringing together software technologies that enable cutting-edge computational science. When we see these amazing results, we may not realize the amount of software infrastructure required to make this research possible and that these achievements were able to be accomplished in amazingly short time frames by judicious use of existing workflow components. None of the Gordon Bell COVID-19 projects used an existing, monolithic workflow system. Rather, each team developed their own tailored workflow solutions, leveraging ExaWorks technologies at key points, to enable scalability while reducing the developer time needed to support the scale and magnitude of these research efforts.”
When we see these amazing results, we may not realize the amount of software infrastructure required to make this research possible and that these achievements were able to be accomplished in amazingly short time frames by judicious use of existing workflow components. None of the Gordon Bell COVID-19 projects used an existing, monolithic workflow system. Rather, each team developed their own tailored workflow solutions, leveraging ExaWorks technologies at key points, to enable scalability while reducing the developer time needed to support the scale and magnitude of these research efforts. – Dan Laney, ExaWorks PI and Computer Scientist in the LLNL Center for Applied Scientific Computing
Award winning remarkable science
The award winning project, AI-Driven Multiscale Simulations Illuminate Mechanisms of SARS-CoV-2 Spike Dynamics, reflects the efforts of a number of scientists at 10 different institutions [i] to incorporate AI into NAMD molecular dynamics (MD) simulations running at scale on the largest leadership supercomputers, such as the Texas Advanced Computing Center (TACC) Frontera [ii] and the GPU-accelerated Summit supercomputer at Oak Ridge National Laboratory (ORNL). To address the challenge of evaluating a potentially huge set of “biologically interesting” conformational changes, along with other achievements in their Gordon Bell paper, the authors created “a generalizable AI-driven workflow that leverages heterogeneous HPC resources to explore the time-dependent dynamics of molecular systems.” [iii] This workflow used DeepDriveMD and components from the ExaWorks SDK.
In the end, the team combined cutting-edge AI techniques with the highly scalable NAMD code to produce a new high watermark for classical MD simulation of viruses by simulating 305 million atoms. The ORNL Summit system was able to deliver impressive sustained NAMD simulation performance, parallel speedup, and scaling efficiency, as shown in the following table (Figure 2) for the full SARS-CoV-2 virion.
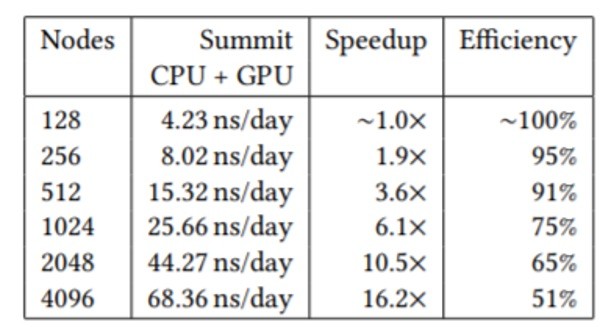
Figure 2: NAMD sustained simulation performance, parallel speedup, and scaling efficiency are reported for the full SARS-CoV-2 vision on Summit
The need for AI
Richard Feynman famously stated that “everything that living things do can be understood in terms of the jiggling and wiggling of atoms.” [iv] The truth of Feynman’s statement is being proven by scientists such as Rommie Amaro, a professor of chemistry and biochemistry at the University of California at San Diego and a contact author on the SC20 COVID-19 award winning paper, who have been able to advance our understanding of how viruses such as COVID-19 operate by leveraging MD simulations.
The SC20 COVID-19 award winning paper is based, in part, on research published in the June 12, 2020, study, Shielding and Beyond: The Roles of Glycans in SARS-CoV-2 Spike Protein. The work was performed by Rommie Amaro and her team, who uncovered surprising information about the exterior shield of the COVID-19 spike protein in the NAMD simulations they performed on the NSF Frontera computing system at the Texas Advanced Computing Center (TACC). Amaro is also the corresponding author of the June 12 study (Figure 3). The spike protein and the lipid membrane to which it is attached is the part of the COVID-19 virus we all try to destroy when we wash our hands for 20 seconds. Contact with soap while we rub our hands destroys the membrane and the ability of the virus to infect cells.
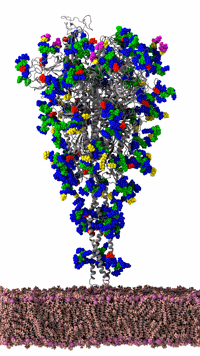
Figure 3: SARS-CoV-2 spike protein of the coronavirus was simulated by the Amaro Lab of the University of California, San Diego on the NSF-funded Frontera supercomputer of the Texas Advanced Computing Center at the University of Texas, Austin. It is the main viral protein involved in host-cell coronavirus infection. Credit: Rommie Amaro, UC, San Diego
Figure 3 shows an animated gif of the spike protein and part of the lipid membrane. While illustrative, the complexity of the molecule and the large number of possible conformations helps us understand why the Gordon Bell COVID-19 award team decided to use AI to target biologically interesting conformational changes. AI helps scientists work smarter because AI can help identify interesting conformational changes that scientists can then simulate and visualize in detail to understand the important molecular changes that occur due to the “jiggling and wiggling of atoms,” as illustrated below and discussed later in this article (Figure 4).
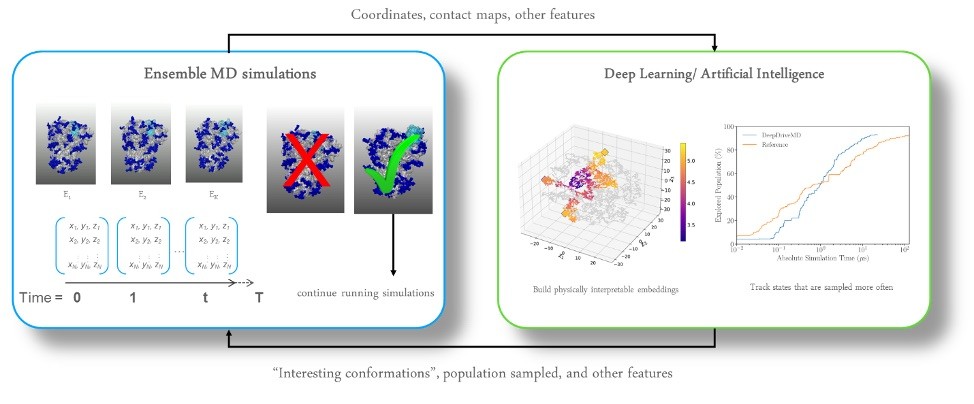
Figure 4: Incorporating AI to target interesting conformational changes that might signal a biologically interesting event
The shield of the spike protein camouflages the virus so it can hide itself from the defending antibodies in our immune system when it enters the body. Surprisingly, the June 12, 2020, study reported that the shield’s sugary coating of molecules, called glycans, also prime the coronavirus to infect a cell by changing the shape of an internal spike protein (Figure 5). “That was really surprising to see,” Amaro noted. “It’s one of the major results of our study. It suggests that the role of glycans in this case is going beyond shielding to potentially having these chemical groups actually being involved in the dynamics (e.g., a conformational changes) of the spike protein,” she said.
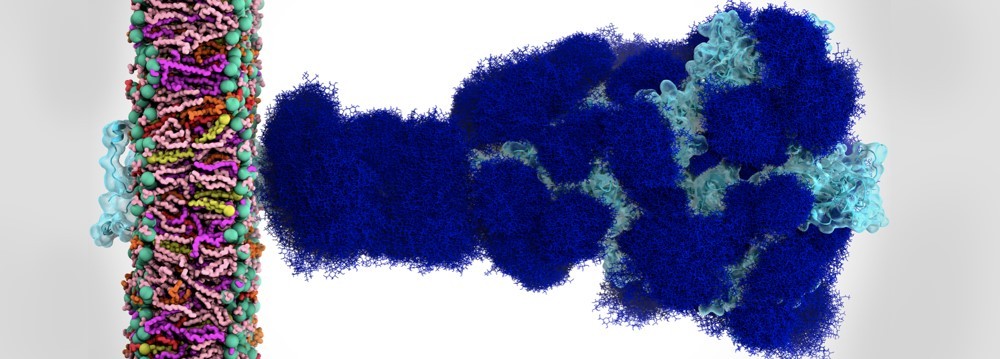
Figure 5: The sugary coating of molecules called glycans (deep blue) that shield the SARS-CoV-2 spike from detection by the human immune system. These glycans change the shape of the spike protein to bind with the ACE2 receptor on human cells. Credit: Lorenzo Casalino et al., UC, San Diego
The inclusion of AI lets scientists work smarter
The challenge is that even with the power of current leadership supercomputers, scientists can still only examine the dynamical behavior of the molecules over very short intervals of time. In addition, for COVID-19, the team wanted to investigate a potentially huge space of conformations not accessible by brute force methods.
The June 12 work did not use AI in the workflow as Amaro’s team focused on behavior the scientists knew was important—specifically the dynamics of the interactions between the spike and the human ACE2 receptor as the virus binds with a human cell to infect it. Such targeted MD simulations have been the mainstay of molecular dynamics research, but can miss important conformational changes that are important to the function of the biological system (Figure 6). The conformational space for even small biological systems like viruses can be quite huge and likely offer many new potential targets for disease treatments and vaccines. For the award-winning SC20 work, the team used the DeepDriveMD (Deep-Learning-Driven Adaptive Molecular Simulations for Protein Folding) toolkit.
DeepDriveMD is an AI-based toolkit jointly developed by a team led by Arvind Ramanathan at Argonne National Laboratory and Shantenu Jha’s team at Rutgers University/ Brookhaven National Laboratory (BNL). [v]
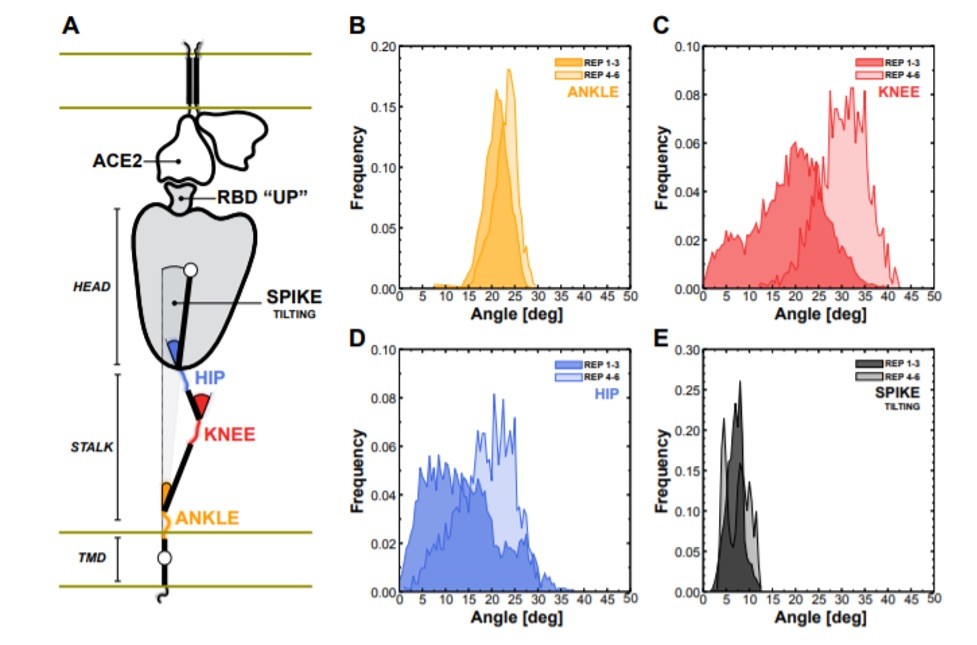
Figure 6: Flexibility of the spike bound to the ACE2 receptor. (A) Schematic representation of the two-parallel-membrane system of the spike-ACE2 complex. (B-E) Distributions of the ankle, knee, hip, and spike-tilting angles resulting from MD replicas 1–3 (darker color) and 4–6 (lighter color). Starting points for replicas 4–6 have been selected using DeepDriveMD, which provided an effective speedup of 1 order of magnitude sampling efficiency (observed 25% more conformations of the knee bending in only 12% of the time).
While not formally a part of the ExaWorks SDK, Shantenu Jha points out that “DeepDriveMD derives its functionality and performance by building upon RADICAL-Cybertools — which is part of the ExaWorks project. We believe this is an excellent validation of the ExaWorks philosophy of a core set of high-performant components that can be easily extended to meet specific requirements, while leaving the performance and workload management and resource management complexity to specialized components.”
DeepDriveMD derives its functionality and performance by building upon RADICAL-Cybertools — which is part of the ExaWorks project. We believe this is an excellent validation of the ExaWorks philosophy of a core set of high-performant components that can be easily extended to meet specific requirements, while leaving the performance and workload management and resource management complexity to specialized components – Shantenu Jha, Computation and Data-Driven Discovery Chair at Brookhaven National Laboratory
Scalable, portable workflow creation subject to tight time constraints
The speed of workflow creation demonstrates the efficacy of the toolkit as it was added after the June 12th publication of the Armaro team paper. Even so, the computer science team was able to generate results in time to complete the computational work so the completed research paper could be delivered in accord with the October 08, 2020, SC20 final paper deadline.
In addition to the tight time constraints, the ExaWorks components were able to meet the scaling requirements of the research competition. Specifically, the research team was able to establish new high watermarks for the classical MD simulation of viruses by simulating 305 million atoms. The team also established a new high watermark for the weighted ensemble method at 600,000 atoms. [vi]
The weighted ensemble method [vii] [viii] runs many short simulations in parallel along a chosen set of reaction coordinates instead of running one single long simulation. This gives scientists the ability to examine conformational changes not accessible by classical MD simulations [ix] as well direct access to both thermodynamic and kinetic properties. [x] The authors note that this gives scientists access to conformational changes that occur on biological timescales generally not accessible by classical MD simulations.
Scalable scheduling enables end-to-end drug design workflows
The Flux resource manager, which is being integrated into the ExaWorks toolbox, was leveraged by another finalist doing drug design. Dong H. Ahn, a computer scientist at LLNL, PI of the Flux project, and one of the Co-PIs of the ExaWorks team, points out the key resource management role Flux performs in the ExaWorks framework. “Flux is a next generation resource manager that helps users create portable high-performance workflows. Flux has been providing the scalable backbone of Livermore’s Rapid COVID-19 Small Molecule Drug Design workflow whose scalable machine-learning work was featured in one of the award finalists.” Flux enabled a multidisciplinary team formed in May 2020 to develop a highly scalable end-to-end drug design workflow that can expediently produce potential COVID-19 drug molecules for further clinical testing. He said, “ExaWorks will provide an unprecedented level of composability of workflow systems in such a way that highly complex workflows like our drug design workflow can be easily architected in a timely fashion.”
Flux will be the resource manager on Livermore’s El Capitan supercomputer, which is projected to be the world’s most powerful supercomputer when it is fully deployed in 2023. [xi] Flux is also portable as it can also be used as a user-space resource manager on systems where Flux is not installed as a system component.
Succinctly, Flux allows co-scheduling of work on modern HPC systems so scientists can run multiple workflow tasks with vastly different time and resource requirements at the same time. Essentially the user creates a DAG (Directed Acyclic Graph) using Parsl or other package like Maestro. Flux can discover resources inside a system to make the best use of resources by concurrently running independent tasks on difference system components like accelerators. The end result is better system utilization and a faster time-to-result.
Swift/T, a high-level programming language to generate workflows
The third finalist, led by researchers at Argonne National Laboratory, adopted workflow technology to develop a highly scalable epidemiological simulation and machine learning (ML) platform. The workflow was a complex structure of CityCOVID, a parallel RepastHPC agent-based modeling simulation of the 2.7 million residents of Chicago[xii], interspersed with batteries of ML-accelerated optimization tasks, all distributed across 4,096 nodes of Argonne’s Intel Xeon Phi-based supercomputer Theta. Managing this workload was enabled through the use of Swift/T, a high-level programming language used by multiple ECP projects, including CANDLE, CODAR, and ExaLearn. Swift/T programs generate workflows that manage execution inside a compute job, reducing the impact on system services including schedulers and the filesystem.
Integrating the complete CityCOVID and ML epidemiological modeling platform was aided by multiple Swift/T design features. The simulation itself is a stand-alone C++ module that, in this case, ran on 256 cores and communicated internally with MPI. Invoking large, concurrent batches of such runs efficiently is one of the main capabilities of the Swift/T runtime, which invoked the simulator repeatedly through library interfaces.
Even more challenging was generating and coordinating the large number of single-node optimization tasks, each of which used vendor-optimized multithreaded math kernels. These single-node tasks were calls to a range of R libraries, dispatched via a custom R parallel backend. Here the work extended the notion of workflow composability, a key theme of the ExaWorks project, into the algorithmic control of the simulation and learning platform through external algorithms developed in “native” ML languages R and Python. This was implemented using the resident, or stateful, task capabilities of Swift/T and the associated EMEWS algorithm control framework, making the development of complex, ML-driven, large-scale workflows more accessible to a wide range of researchers across scientific domains. Additionally, the workflow recorded progress in a SQL database, accessed via workflow tasks expressed in the Python language, also bundled with Swift/T.
“This was an opportunity to demonstrate the impact of workflow research on a large-scale, critical problem,” Justin Wozniak (lead of the Swift/T workflow system) said. “It was great to see that a reusable technology developed for exascale could have an impact on a pressing real-world application.”
A key point made in the Gordon Bell nomination was the conceptual re-architecturing of the high-performance computing problem. Rather than being an isolated number cruncher, Theta was brought into a dynamic data pipeline, consuming real-world infection data and producing database records for distribution to stakeholders at the city and state level. As described in a letter of reference, City of Chicago Department of Public Health (CPDH) Commissioner Dr. Allison Arwady said, “CDPH has used your modeling way to explain outbreak modeling to residents, government officials, and many other stakeholders… research that has been invaluable to our citywide planning efforts and integral for decision making.” More broadly, her endorsement demonstrated that such data and simulation pipelines will have broad applicability as big data analysis workloads are combined with high-performance simulation and learning; as she stated that this work “has the potential to make impacts well beyond COVID-19, to a general rapid response scientific platform.”
“Through our novel integration of machine learning and agent-based simulation modeling, we have demonstrated how science can more closely support decision makers for crisis response and planning. This workflow-technology-enabled approach will be able to exploit future hybrid exascale architectures, for interdependent CPU and GPU based tasks, to further facilitate evidence-based decision making,” said Argonne computational scientist Jonathan Ozik.
Summary
Kudos to the Gordon Bell COVID-19 winner and finalists! Not only did these researchers provide scientific insight to advance our knowledge of a deadly disease that is currently destroying lives and economies around the world but they also helped validate the behind-the-scenes efforts of the computer scientists who created DeepDriveMD and the ExaWorks SDK. Brookhaven has a nice write-up of DeepDriveMD on the web.
Funded in part by the ECP, the ExaWorks SDK is designed to enable a portable and sustainable software infrastructure for workflows that can be used by many projects. The intent is to facilitate a convergence in the software workflows community.
Community engagement is the key to the success of the ExaWorks project. The intent is to begin to harmonize the disparate workflow landscape and ensure ExaWorks components operate on current and future systems. The success of the high-profile Gordon Bell COVID-19 award projects that used ExaWorks technologies is a clear indication that the core ExaWorks strategy of easily adopted, composable workflow components provides a path to performant and portable exascale workflows for the HPC community.
Rob Farber is a global technology consultant and author with an extensive background in HPC and in developing machine learning technology that he applies at national laboratories and commercial organizations. Rob can be reached at [email protected]
[i] University of California San Diego, Argonne National Lab, University of Illinois at Urbana-Champaign, University of Pittsburgh, Rutgers University & Brookhaven National Lab, University of Southampton, Stony Brook University, NVIDIA Corporation, Texas Advanced Computing Center, San Diego Supercomputing Center
[ii] https://www.tacc.utexas.edu/-/coronavirus-massive-simulations-completed-on-frontera-supercomputer
[iii] SC’20: International Conference for High Performance Computing, Networking, Storage, and Analysis. ACM, New York, NY, USA
[iv] https://www.americanscientist.org/article/wiggling-and-jiggling#:~:text=phase%2Dspace%20perspective.-,As%20Richard%20Feynman%20famously%20said%2C%20%E2%80%9Ceverything%20that%20living%20things%20do,the%20probable%20locations%20of%20electrons).
[v] https://arxiv.org/pdf/1909.07817.pdf
[vi] The authors note the barnase-barnstar complex with 100,000 atoms was the previous high water mark.
[vii] https://www.ncbi.nlm.nih.gov/pmc/articles/PMC1224912/
[viii] https://www.annualreviews.org/doi/10.1146/annurev-biophys-070816-033834
[ix] https://www.annualreviews.org/doi/10.1146/annurev.physchem.48.1.545
[x] https://aip.scitation.org/doi/10.1063/1.3306345
[xi] https://www.llnl.gov/news/llnl-and-hpe-partner-amd-el-capitan-projected-worlds-fastest-supercomputer
[xii] https://www.anl.gov/article/researchers-use-alcf-resources-to-model-the-spread-of-covid19