Additive manufacturing (AM), also known as 3D Printing, is revolutionizing manufacturing by enabling construction of complex parts that cannot be readily fabricated by traditional means. Although most people are familiar with desktop 3D printers that can create small objects by melting plastic, it is also possible to create metal parts (e.g., titanium, stainless steel) using similar techniques. One of the most exciting aspects of metal AM is creating materials tailored to specific applications by adjusting process-control variables to achieve desired physical properties that might even vary throughout a given part.
Technical Introduction
In AM, a geometric description of the part is processed into 2D slices, a feedstock material is melted, and the part is built layer-by-layer. In metal AM, the feedstock is often in wire or powder form, and the energy source is a laser or electron beam. The Exascale AM project (ExaAM) focuses on powder bed processes, in which each layer is approximately 50 microns. So, a part that is 1 cm tall requires about 200 layers, with each layer consisting of new feedstock powder and one or more passes of a laser or electron beam to sinter and/or melt the powder in appropriate locations.
A complex interplay between physical phenomena determines the performance of the final manufactured part. Fortunately, these phenomena are directly influenced by controllable process parameters, such as the pattern by which each layer is melted, the diameter, magnitude, and speed of the energy source. In turn, these parameters influence the microstructure throughout the part, which determines local properties and ultimately the ability of the object to perform its intended role. This sequence is often referred to as the process-structure-property-performance (PSPP) relationship. There are significant gaps in understanding this relationship for AM processes; filling these gaps where possible and quantifying uncertainties where it is not are key to unlocking the potential of AM.
The physical processes involved in AM are similar to those of welding—a field with a wealth of experimental, modeling, simulation, and characterization research knowledge. Unfortunately, the simulation tools developed for welding and other similar processes, although well calibrated and approaching predictive capability, are inadequate for AM processes as demonstrated by the inability to predict AM build failures for new designs. We believe that this is largely because the PSPP relationship is traditionally modeled in an uncoupled manner, thereby relying on tabular databases that are unable to adequately capture the implicit, dynamic, non-equilibrium nature of AM processes.
One of the goals of ExaAM is to remove those limitations by coupling high-fidelity mesoscale simulations within continuum process simulations to determine microstructure and properties using local conditions. To that end, the ExaAM team is developing a suite of exascale-ready computational tools that operate in a sequential multistage workflow. By simulating the microstructure created by melting and solidifying the metal powder, we can predict how the material will behave when used. By leveraging exascale computing platforms, ExaAM can predict the microstructure and properties and quantify their uncertainties (i.e., determine our confidence in the predictions). Knowing this is critical to qualifying and ultimately certifying parts for specific applications. John Turner (PI of the ExaAM project and Computational Engineering Program director at Oak Ridge National Laboratory [ORNL]) notes that “accurate, high-fidelity simulations can provide the confidence in our designs and processes that we need to qualify AM parts for a range of applications.”
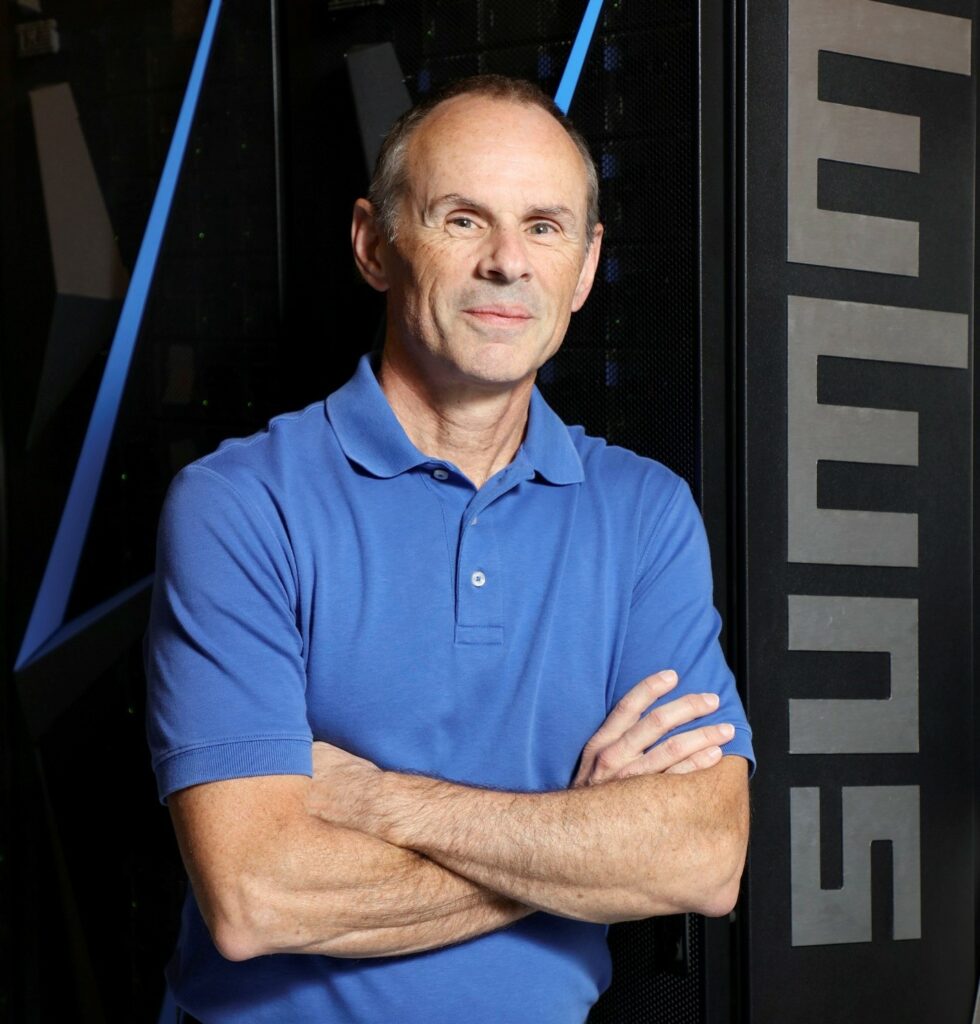
Figure 1. John Turner, PI of the ExaAM project and Computational Engineering Program director at ORNL
Technology Brief
AM began in the 1980s as a means of rapidly creating prototypes rather than for manufacturing final parts. Over the next few decades, the concepts and processes of AM matured, and in the early to mid 2000s, several things happened that led to a rapid expansion of the technology.
First, some of the early patents expired, thereby opening the door for inexpensive machines from multiple vendors. Then, the sensors and control systems used in AM became more sensitive and more advanced. For example, think of the sensors in your phone that enable it to determine whether you’re holding it horizontally or vertically. Finally, computational capabilities also increased dramatically in this timeframe. All these factors contributed to the rapid expansion of AM technologies.
The most visible expansion occurred in the consumer space with the arrival of very affordable desktop machines for making polymer plastic parts. These machines would partially melt plastic polymer and extrude it—push it out—through a nozzle to build whatever part you were making.
Less visible were the dramatic strides in 3D printing of metal parts. The systems to make metal parts are much more complex and expensive and thus not as accessible to consumers. However, these innovations have the potential to impact manufacturing and supply chains to a much larger degree.
Most of these 3D printing processes—for both plastic and metal—involve building a 3D model of an object in computer software, using that software to mathematically slice the object into many 2D layers, and then building the actual physical part layer-by-layer. For metals, there are several different AM processes, all of which involve feedstock material (e.g., powder, wire) and an energy source (e.g., laser, electron beam).
An Expanded Design Space
One of the main advantages of AM is the expansion of the design space, which results in increased flexibility to optimize the process for specific uses. That also includes the material choice—titanium, stainless steel, and other nickel-based alloys can be used in metal AM. You can even blend materials to create hybrids with unique properties. One of the most exciting aspects of AM is the ability to control the characteristics of different sections of a single part.
Consider a bicycle frame. The distribution of stresses varies dramatically between the top tube, the bottom bracket, and the fork, and those stresses depend on use. The stresses of a leisurely ride around the neighborhood are dramatically different from those a racer experiences on a stage of the Tour de France. In addition, there is a big difference between a time trial, in which the cyclist delivers steady, consistent power over the course of an hour or so, and an attack on a steep mountain climb where the cyclist is out of the saddle. You can imagine there are very different stresses at different locations throughout a bicycle frame.
Over the years, the design of bicycle frames has evolved based on those stresses and failure points. With AM, we can adjust the parameters used in the process (e.g., beam speed, power, path) and obtain different properties at different locations on the part. Ideally, we use this information within the design process to obtain a design that is optimized for a particular use. For example, we could optimize for a racing bike by minimizing the weight and optimize for a recreational bike by minimizing the cost and maximizing the lifetime. There is much more design flexibility in the additive process.
One major challenge is the complex interplay between the physical phenomena (e.g., heat transfer, melting, solidification, fluid flow) involved in the additive process. The capabilities developed by ExaAM are improving our understanding of those processes, how they interact, and how they affect each other. This understanding is required to achieve optimal designs in the design phase itself—before expensive prototyping—and to qualify AM parts for critical applications, including use in motor vehicles and aerospace.
For more information, see:
- A. Turner, J. Belak, N. Barton, M. Bement, N. Carlson, R. Carson, S. DeWitt, J.-L. Fattebert, N. Hodge, Z. Jibben, W. King, L. Levine, C. Newman, A. Plotkowski, B. Radhakrishnan, S. T. Reeve, M. Rolchigo, A. Sabau, S. Slattery, and B. Stump, “ExaAM: Metal additive manufacturing simulation at the fidelity of the microstructure,” International Journal of High Performance Computing Applications 36, no. 1 (January 2022): 13–39, https://doi.org/10.1177/10943420211042558.
- Plotkowski, J. Ferguson, B. Stump, W. Halsey, V. Paquit, C. Joslin, S. S. Babu, A. Marquez Rossy, M. M. Kirka, and R. R. Dehoff, “A stochastic scan strategy for grain structure control in complex geometries using electron beam powder bed fusion,” Additive Manufacturing 46 (October 2021): 102092, https://doi.org/10.1016/j.addma.2021.102092.
Progress to date
A significant challenge for simulating AM processes is that there is no single software tool that can capture all the relevant physics required. However, several applications have been developed within the US Department of Energy complex and in the broader computational science community to simulate phenomena similar to those required for AM. ExaAM is leveraging several of those existing capabilities by enhancing and extending them as needed and developing new capabilities when necessary.
For example, we extended the widely used OpenFOAM open-source computational fluid dynamics tool to perform melt pool thermal simulations with additiveFOAM. A new cellular automata capability has been developed for microstructure evolution in the ExaCA program. We also leveraged other Exascale Computing Project (ECP) efforts to create new exascale-ready, AM-specific capabilities. For example, our polycrystal plasticity capability for micromechanical properties effort, known as ExaConstit, is built on MFEM.
These physics components are executed in a sequence of linked simulations depending on the question at hand. For example, one of our standard problems involves predicting strain in a test part that is made of Inconel 625, which is a widely used nickel-based superalloy. For this study, we use the sequence of simulations shown in Figure 2.
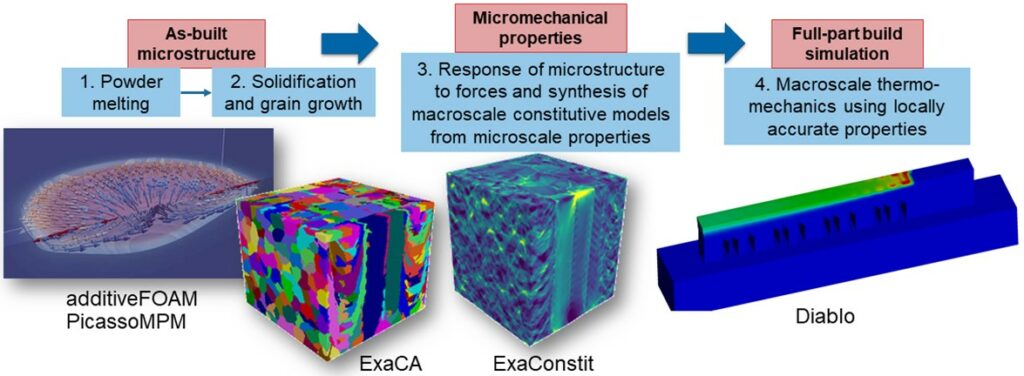
Figure 2. Sequence of physics simulations required to predict the microstructure, properties, and strain field of an AM Inconel 625 part.
Table 1 provides more information about the physics components used in each of the stages. These components are supported and extended by components shown in Table 2.
Table 1. Core ExaAM physics components used to predict the microstructure, properties, and strain field of an AM Inconel 625 part.
Stage | Description | Component(s) | Location |
1 | Melting | additiveFOAM PicassoMPM |
Not yet released https://github.com/ECP-copa/ExaMPM |
2 | Solidification and grain growth | ExaCA | https://github.com/LLNL/ExaCA |
3 | Micromechanical properties | ExaConstit | https://github.com/LLNL/ExaConstit |
4 | Locally accurate, process-informed, full-part build simulation | Diablo | Export Controlled (EAR99) |
Table 2. Additional ExaAM physics components used to inform and improve core components and for expanded scientific inquiry.
Description | Component(s) | Location |
Subgrain microstructure | AMPE Tusas |
https://github.com/LLNL/AMPE https://github.com/chrisknewman/tusas |
Solidification with subscale fluid flow | iAMCool | Not yet released |
Late-time microstructure | MEUMAPPS-SS | https://github.com/ORNL/meumapps_ss |
In addition to developing and extending the physics capabilities of these components, we have also made significant progress in optimizing them for exascale platforms. Our emphasis is on the most computationally intensive components, such as ExaCA, ExaConstit, ExaMPM, and Tusas, but all components are running on early access platforms such as Crusher to ensure that they will be ready for systems such as Frontier.
Another unique aspect of the ExaAM project is our emphasis on enabling these capabilities to execute in a connected manner. This integration results in improved accuracy throughout the additive process—from melting to solidification and microstructure evolution to property prediction. ExaAM is collaborating with the ExaWorks project for this aspect of development.
Uncertainty Quantification
Owing to the complex nature of ExaAM simulations and their dependence on inexact data (e.g., geometry, material properties, mechanical tolerances), some uncertainty in the results is inevitable. To qualify parts, an understanding of the amount of uncertainty is critical (i.e., essentially error bars on simulation results).
ExaAM is using TASMANIAN (https://tasmanian.ornl.gov/), which is another ECP-supported effort and part of the ALExa Software Technologies project, to intelligently sample key parameters over a range of values, thus enabling a quantification of the uncertainty in the final results—uncertainty caused by inexact information about those parameters. We are also collaborating with ExaWorks to automate the ExaAM workflow to enable uncertainty quantification and embedding in design optimization loops.
Summary
The ExaAM toolkit is coupling multiple physics applications to enable an exascale-capable, multiscale, multiphysics simulation toolkit that can be used to accelerate the certification of AM processes.
This research was supported by the Exascale Computing Project (17-SC-20-SC), a joint project of the US Department of Energy’s Office of Science and the National Nuclear Security Administration, responsible for delivering a capable exascale ecosystem, including software, applications, and hardware technology, to support the nation’s exascale computing imperative.